Combining knowledge for eHealth solutions
A typical eHealth scenario revolves around a patient or user group suffering from a chronic condition. Our goal is to develop tools that can help users to self-manage their condition, or even to prevent or delay the condition from arising, thus limiting his dependency on professional healthcare. To achieve this, we combine the knowledge and experience from all required disciplines at the University of Twente, such as biomedical engineering, computer science and behavioral science.
There are five key research lines that need to be mastered in order to deliver successful personalized eHealth solutions.
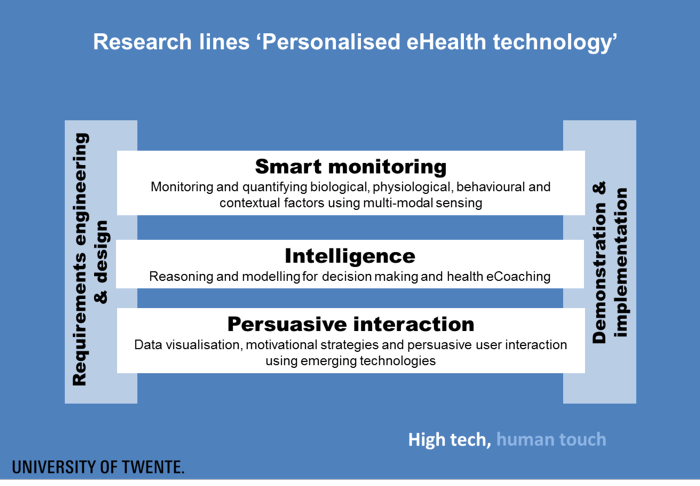
Research lines
Requirements Engineering & Design A crucial first step on the path to a successful personalised eHealth solution is understanding the needs and desires of the target users – typically a primary user or patient and the stakeholders surrounding the user – such as healthcare professionals, informal caregivers and the user’s social environment. For the context of personalised eHealth technology, these activities should cover functional design, as well as the development of associated care protocols or service models. Research activities will involve:
- Investigate new design research approaches that do not rely exclusively on asking what people would like to have/use, but use innovative methods for example through gamifying requirements elicitation sessions. At the same time, develop and validate online tools that allow for online requirements elicitation, but that do foster the same atmosphere as can be created in face-to-face meetings, allowing to gather requirements from a group of diverse people that are geographically dispersed and difficult to get together.
- Developing a taxonomy for classifying requirements for eHealth, and subsequently creating a platform for distributing them should enable a huge gain in efficiency while engineering requirements.
- Incorporate the issue of ‘trust’ as a critical point to the count on high end-user acceptance. But at the moment, our knowledge of the coming about and workings of trust with respect to personalised eHealth technology are very limited.
Smart Monitoring and Multimodal Sensing Any personalised eHealth solution will involve gathering of data from various sources and with different characteristics. The first level ‘building block’ therefore consists of development and usage of technologies that help us understand the user (typically focusing on the primary user/patient) and quantify his biological, physiological, behavioural and contextual factors. The challenge of the monitoring is to obtain a holistic view on the functioning of the person in his/her context. This will involve several layers of sensing, ranging from sensing of biological and physiological processes, up to more behavioural aspects involving social, cognitive aspects, subjective experiences on health and wellness and contextual information to support a proper interpretation of all health and wellness aspects. The sensor data characteristics are basically very different in their characteristics, not only in their scales but also with respect to their sampling rates. Feature engineering will be needed to create homogeneous longitudinal datasets that enable further analysis. It is not our intention to develop sensors themselves, but seeking collaboration with experts in this area, like the sensor program at UTwente and use state of the art sensing technology commercially available. Major challenges that we will address will involve:
- Creating models and approaches of data gathering, that allow us to create holistic maps of physical, cognitive, social, emotional, and environmental parameters in relationship to the person’s health or wellbeing;
- Develop and apply new feature engineering technology to extract and synchronise the key variables in streaming data sets, reflecting key health and wellness aspects at group and individual level.
- Integrate new on-body or in-body sensor technology with our holistic approach to gain new knowledge on their interaction and create new models on monitoring of diseases.
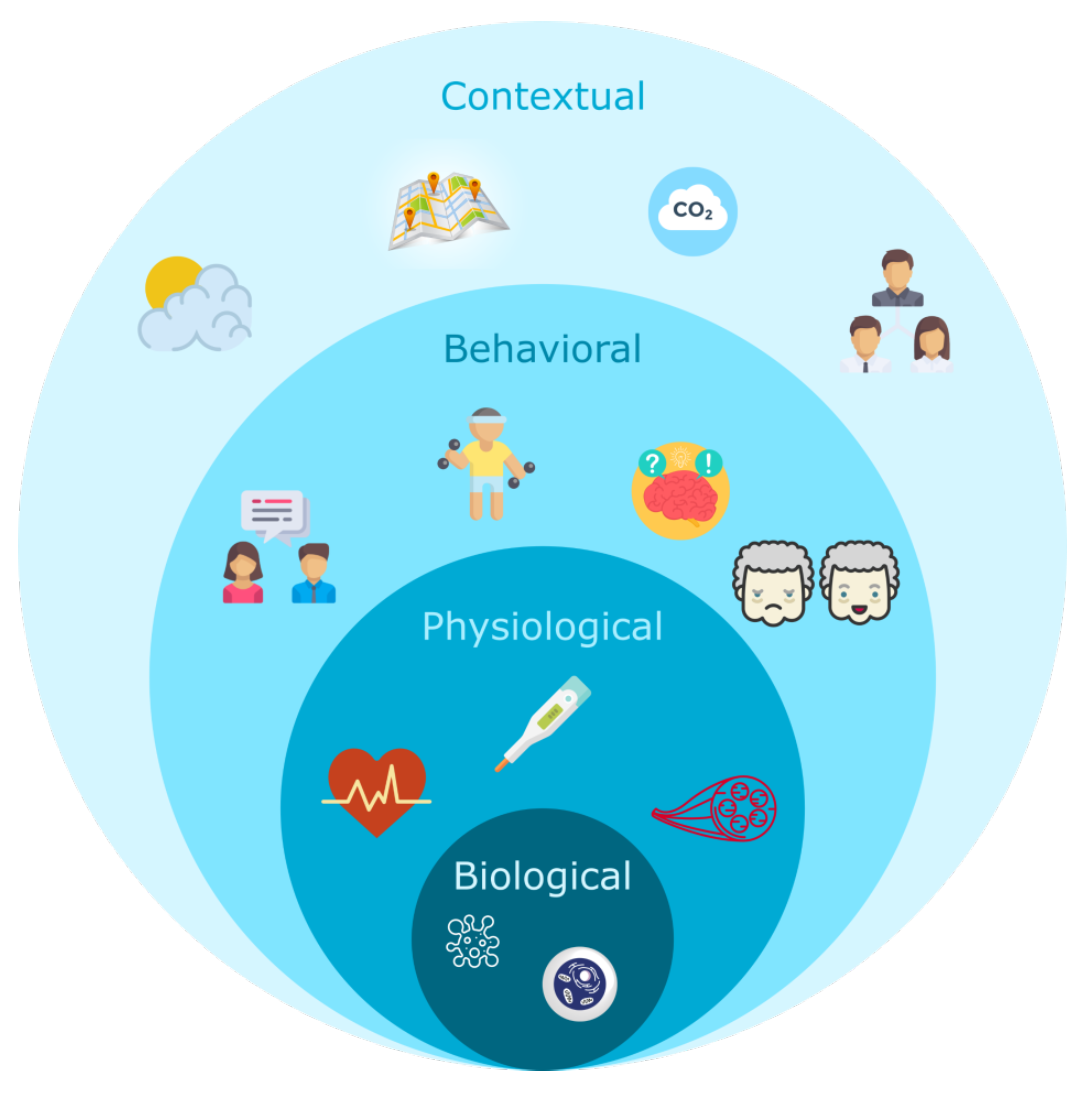
Figure: layers of sensing
Intelligence for Decision making & Coaching Whereas smart monitoring and sensing provides the first level of interpretation of sensor data – additional reasoning is often required to feed the user interfaces that enable the interaction with the various user groups. As a central building block, intelligence and decision making provides the bridge between sensing and interaction. In this research line, we will be dealing with (i) high level semantically meaningful concepts, (ii) deriving information post-hoc from multiple data sources, and (iii) defining fluent models of users or context. Major challenges that we will address involve:
- Creating holistic models that allow us to understand the intricate complexities of interactions between physical, cognitive, social, emotional, and environmental parameters in relationship to the person’s health or wellbeing goals;
- Defining and constructing models of users or health status in ways that foster interoperability in a rapidly digitizing environment;
- Designing the digital mind of the coach of the future – paving the way for truly natural and personalised interactions between virtual coaches and users;
- Creating open, collaborative platforms for the collection, storage, and analysis of large scale heterogeneous multi-modal data;
- Integrating the rapidly advancing technologies in the area of data science, deep learning and artificial intelligence in order to make optimal use of the wealth of data collected.
This building block concerns the design and development of user interaction tools, strategies and visualisations. The underlying knowledge about the user, goals, motivational regulations, and context is translated into concrete interactive tools that are tailored to the end-user in a motivating, understandable and engaging manner. The persuasive interaction line deals with building the right tools for the specified user, on a given interaction device, translating the desired intention into successful interaction strategies. We will address the following key challenges in this line of research:
- Derive fundamental knowledge-base for device- and technology-agnostic personalised user interaction and persuasive communication;
- Design and develop efficient and usable interactive tools using emerging technologies such as virtual reality, holograms, and future interactive technologies;
- Create persuasive and steering interaction strategy models to understand the interaction between motivation strategies for behaviour change and persuasive strategies for engagement and adherence;
- Create persuasive profiles, using data and real-time strategies (Just in time adaptive interventions to change behaviour);
- Develop methods to link the personal preferences, besides attitude or different physical capabilities, of any target group into a specific model for persuasive interaction design.
Demonstration, Evaluation & Implementation studies User studies are a vital part of creating successful personalised eHealth applications throughout the design and development of the technology, as well as to provide evidence needed for successful implementation into daily practice. Through iterative design and continuous user evaluations, design decisions can be validated as the eHealth solution matures. As eHealth solutions are both complex and disruptive, innovative evaluation methods are required to effectively generate evidence needed for implementation into daily care practices. Key challenges of this research line are:
- Development of iterative evaluation approaches, testbeds and methodologies that fit the iterative design approach and grow with the maturity of the technology;
- Development of automated new outcome criteria coming from the sensing and end user interaction modules;
- Research into implementation scenarios and behavioural change strategies to ensure real uptake in by users in daily practice;
- Development of business modelling methods and tools that give insight in the revenues and costs for the whole pallet of stakeholders involved as well as for each of them involved, separately.
Translational research
In our research, we aim to cover the whole chain from development towards contributing to sustainable implementation in daily care. The environment in which technology is being co-developed with end-users and other stakeholders can be described in three categories:
- Living lab type 1: the environment where the first experiments with technology take place to show and test working principles. Often with students and a loose experimental set-up. Examples are the labs of the research groups that are involved in the eHealth programme.
- Living Lab type 2: a lab where reality is simulated to test the technology in a realistic setting but in a safe and very controlled environment. An example is the eHealth house that is being realized in the TechMed Centre.
- Living Lab type 3: technology is used in the real-life, the natural environment where people live and do their things of daily living. We realize these environments in cooperation with our external partners.
We aim to cover the whole translational chain from development towards contributing to sustainable implementation in daily care.
Figure: translational research, from the first experiments to implementation in real life. Click for a larger version.